Theoretical Paper
- Computer Organization
- Data Structure
- Digital Electronics
- Object Oriented Programming
- Discrete Mathematics
- Graph Theory
- Operating Systems
- Software Engineering
- Computer Graphics
- Database Management System
- Operation Research
- Computer Networking
- Image Processing
- Internet Technologies
- Micro Processor
- E-Commerce & ERP
Practical Paper
Industrial Training
Fourier transforms
brief description
the fourier transform is an important image processing tool which is used to decompose an image into its sine and cosine components. the output of the transformation represents the image in the fourier or frequency domain, while the input image is the spatial domain equivalent. in the fourier domain image, each point represents a particular frequency contained in the spatial domain image.
the fourier transform is used in a wide range of applications, such as image analysis, image filtering, image reconstruction and image compression.
how it works
as we are only concerned with digital images, we will restrict this discussion to the discrete fourier transform (dft).
the dft is the sampled fourier transform and therefore does not contain all frequencies forming an image, but only a set of samples which is large enough to fully describe the spatial domain image. the number of frequencies corresponds to the number of pixels in the spatial domain image, i.e. the image in the spatial and fourier domain are of the same size.

where f(a,b) is the image in the spatial domain and the exponential term is the basis function corresponding to each point f(k,l) in the fourier space. the equation can be interpreted as: the value of each point f(k,l) is obtained by multiplying the spatial image with the corresponding base function and summing the result.
the basis functions are sine and cosine waves with increasing frequencies, i.e. f(0,0) represents the dc-component of the image which corresponds to the average brightness and f(n-1,n-1) represents the highest frequency.
in a similar way, the fourier image can be re-transformed to the spatial domain. the inverse fourier transform is given by:

note the normalization term in the inverse transformation. this normalization is sometimes applied to the forward transform instead of the inverse transform, but it should not be used for both.$$
to obtain the result for the above equations, a double sum has to be calculated for each image point. however, because the fourier transform is separable, it can be written as
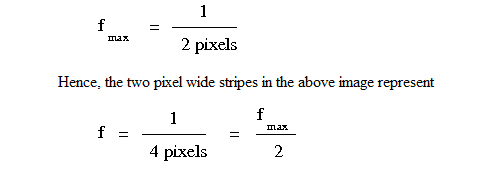
using these two formulas, the spatial domain image is first transformed into an intermediate image using n one-dimensional fourier transforms. this intermediate image is then transformed into the final image, again using n one-dimensional fourier transforms. expressing the two-dimensional fourier transform in terms of a series of 2n one-dimensional transforms decreases the number of required computations.
even with these computational savings, the ordinary one-dimensional dft has complexity. this can be reduced to if we employ the fast fourier transform (fft) to compute the one-dimensional dfts. this is a significant improvement, in particular for large images. there are various forms of the fft and most of them restrict the size of the input image that may be transformed, often to where n is an integer. the mathematical details are well described in the literature.
the fourier transform produces a complex number valued output image which can be displayed with two images, either with the real and imaginary part or with magnitude and phase. in image processing, often only the magnitude of the fourier transform is displayed, as it contains most of the information of the geometric structure of the spatial domain image. however, if we want to re-transform the fourier image into the correct spatial domain after some processing in the frequency domain, we must make sure to preserve both magnitude and phase of the fourier image.
the fourier domain image has a much greater range than the image in the spatial domain. hence, to be sufficiently accurate, its values are usually calculated and stored in float values.
The magnitude calculated from the complex result is shown in
We can see that the DC-value is by far the largest component of the image. However, the dynamic range of the Fourier coefficients (i.e. the intensity values in the Fourier image) is too large to be displayed on the screen, therefore all other values appear as black. If we apply a logarithmic transformation to the image we obtain
The result shows that the image contains components of all frequencies, but that their magnitude gets smaller for higher frequencies. Hence, low frequencies contain more image information than the higher ones. The transform image also tells us that there are two dominating directions in the Fourier image, one passing vertically and one horizontally through the center. These originate from the regular patterns in the background of the original image. The phase of the Fourier transform of the same image is shown in
The value of each point determines the phase of the corresponding frequency. As in the magnitude image, we can identify the vertical and horizontal lines corresponding to the patterns in the original image. The phase image does not yield much new information about the structure of the spatial domain image; therefore, in the following examples, we will restrict ourselves to displaying only the magnitude of the Fourier Transform.
Before we leave the phase image entirely, however, note that if we apply the inverse Fourier Transform to the above magnitude image while ignoring the phase (and then histogram equalize the output) we obtain
Although this image contains the same frequencies (and amount of frequencies) as the original input image, it is corrupted beyond recognition. This shows that the phase information is crucial to reconstruct the correct image in the spatial domain.
We will now experiment with some simple images to better understand the nature of the transform. The response of the Fourier Transform to periodic patterns in the spatial domain images can be seen very easily in the following artificial images.
stp2fur1
If we look carefully, we can see that it contains 3 main values: the DC-value and, since the Fourier image is symmetrical to its center, two points corresponding to the frequency of the stripes in the original image. Note that the two points lie on a horizontal line through the image center, because the image intensity in the spatial domain changes the most if we go along it horizontally.
The distance of the points to the center can be explained as follows: the maximum frequency which can be represented in the spatial domain are two pixel wide stripe pairs (one white, one black).
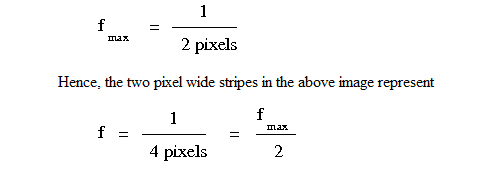
Thus, the points in the Fourier image are halfway between the center and the edge of the image, i.e. the represented frequency is half of the maximum.
Further investigation of the Fourier image shows that the magnitude of other frequencies in the image is less than Eqn:eqnfoura of the DC-value, i.e. they don't make any significant contribution to the image. The magnitudes of the two minor points are each two-thirds of the DC-value.
Similar effects as in the above example can be seen when applying the Fourier Transform
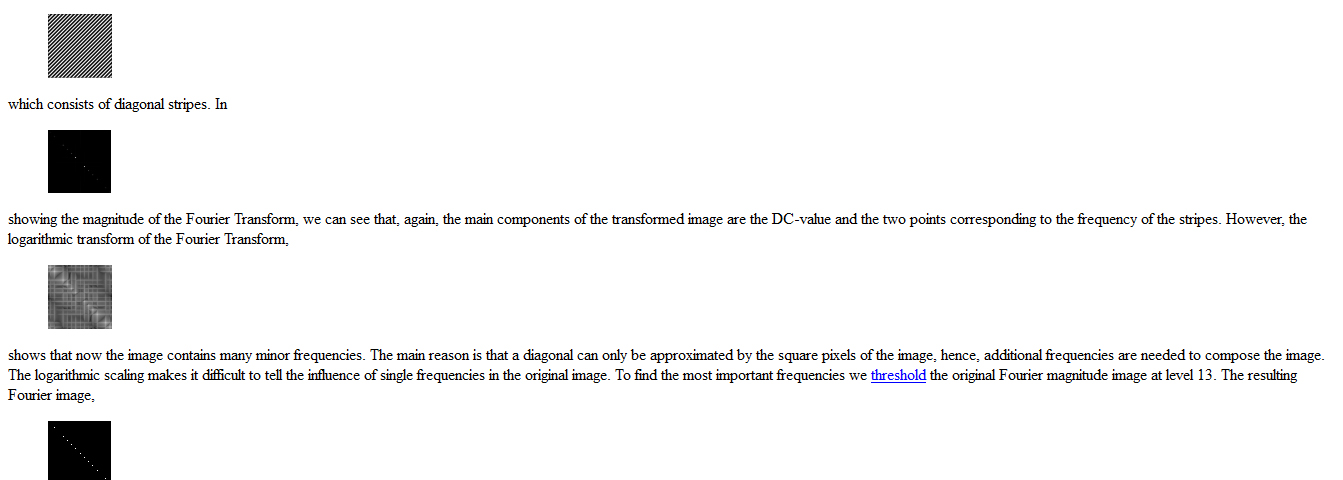
shows all frequencies whose magnitude is at least 5% of the main peak. Compared to the original Fourier image, several more points appear. They are all on the same diagonal as the three main components, i.e. they all originate from the periodic stripes. The represented frequencies are all multiples of the basic frequency of the stripes in the spatial domain image. This is because a rectangular signal, like the stripes, with the frequency Eqn:eqnfourb is a composition of sine waves with the frequencies Eqn:eqnfourc, known as the harmonics of Eqn:eqnfourb. All other frequencies disappeared from the Fourier image, i.e. the magnitude of each of them is less than 5% of the DC-value.
A Fourier-Transformed image can be used for frequency filtering. A simple example is illustrated with the above image. If we multiply the (complex) Fourier image obtained above with an image containing a circle (of r = 32 pixels), we can set all frequencies larger than Eqn:eqnfourb to zero as shown in the logarithmic transformed image
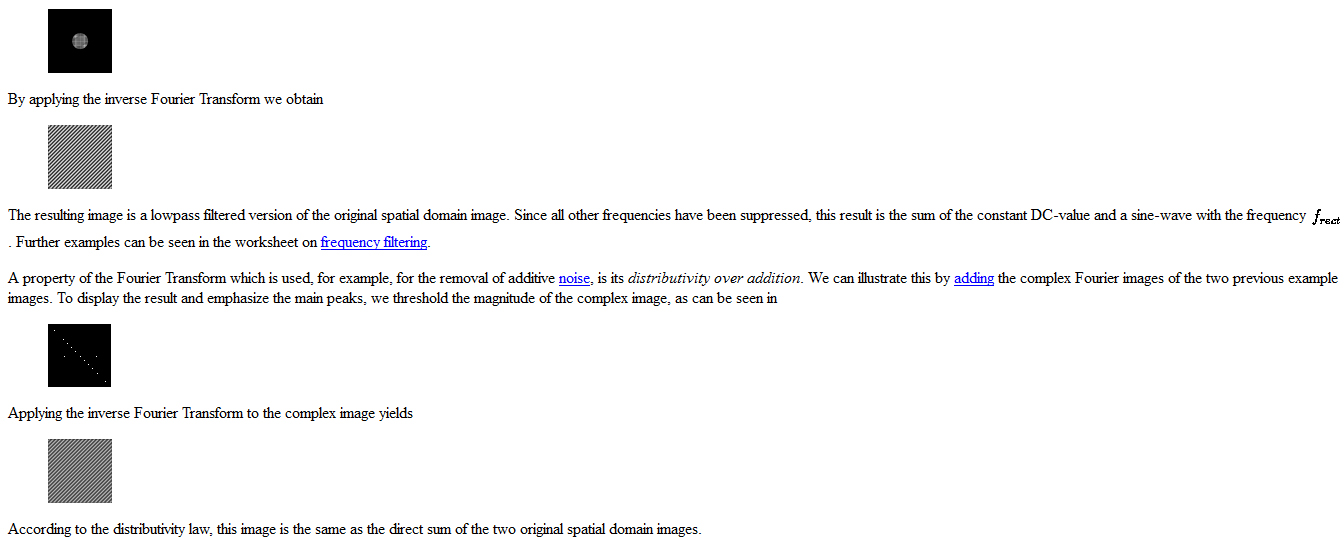
According to the distributivity law, this image is the same as the direct sum of the two original spatial domain images. Finally, we present an example (i.e. text orientation finding) where the Fourier Transform is used to gain information about the geometric structure of the spatial domain image. Text recognition using image processing techniques is simplified if we can assume that the text lines are in a predefined direction. Here we show how the Fourier Transform can be used to find the initial orientation of the text and then a rotation can be applied to correct the error. We illustrate this technique using
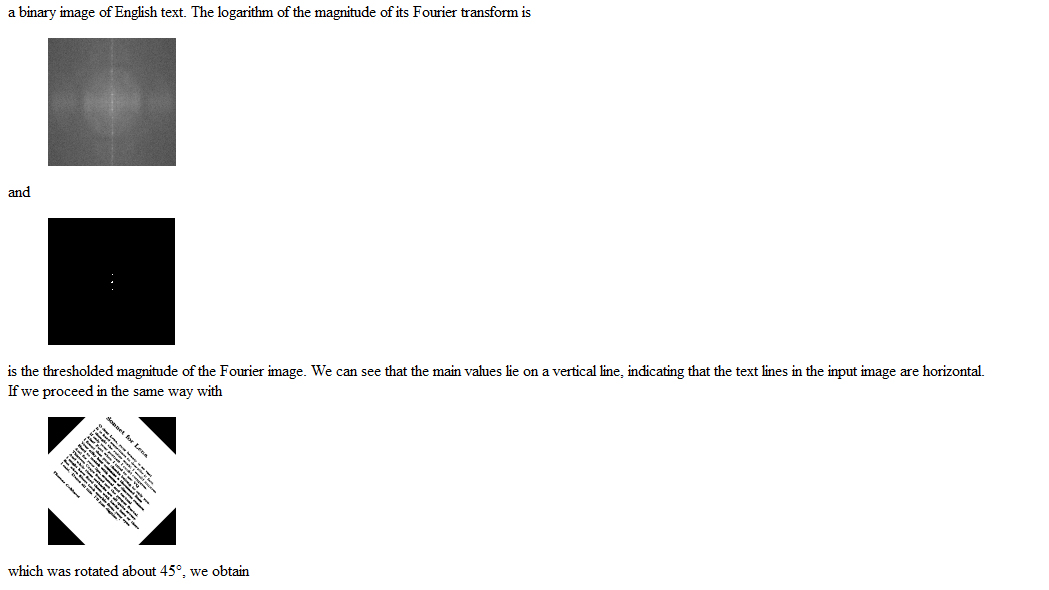
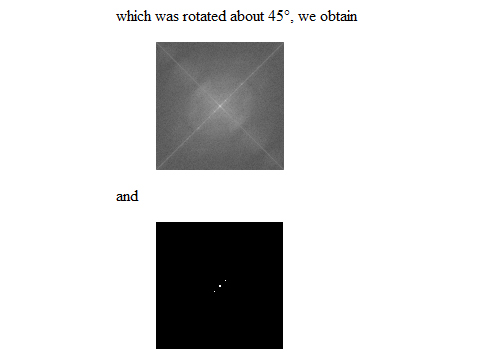
in the Fourier space. We can see that the line of the main peaks in the Fourier domain is rotated according to rotation of the input image. The second line in the logarithmic image (perpendicular to the main direction) originates from the black corners in the rotated image.
Although we managed to find a threshold which separates the main peaks from the background, we have a reasonable amount of noise in the Fourier image resulting from the irregular pattern of the letters. We could decrease these background values and therefore increase the difference to the main peaks if we were able to form solid blocks out of the text-lines. This could, for example, be done by using a morphological operator.
Common Variants
Another sinusoidal transform (i.e. transform with sinusoidal base functions) related to the DFT is the Discrete Cosine Transform (DCT). For an N×N image, the DCT is given by
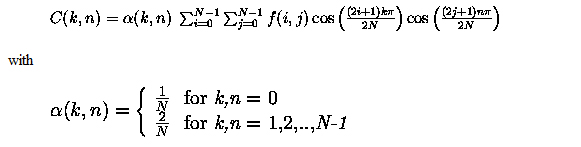
The main advantages of the DCT are that it yields a real valued output image and that it is a fast transform. A major use of the DCT is in image compression --- i.e. trying to reduce the amount of data needed to store an image. After performing a DCT it is possible to throw away the coefficients that encode high frequency components that the human eye is not very sensitive to. Thus the amount of data can be reduced, without seriously affecting the way an image looks to the human eye.
Exercises
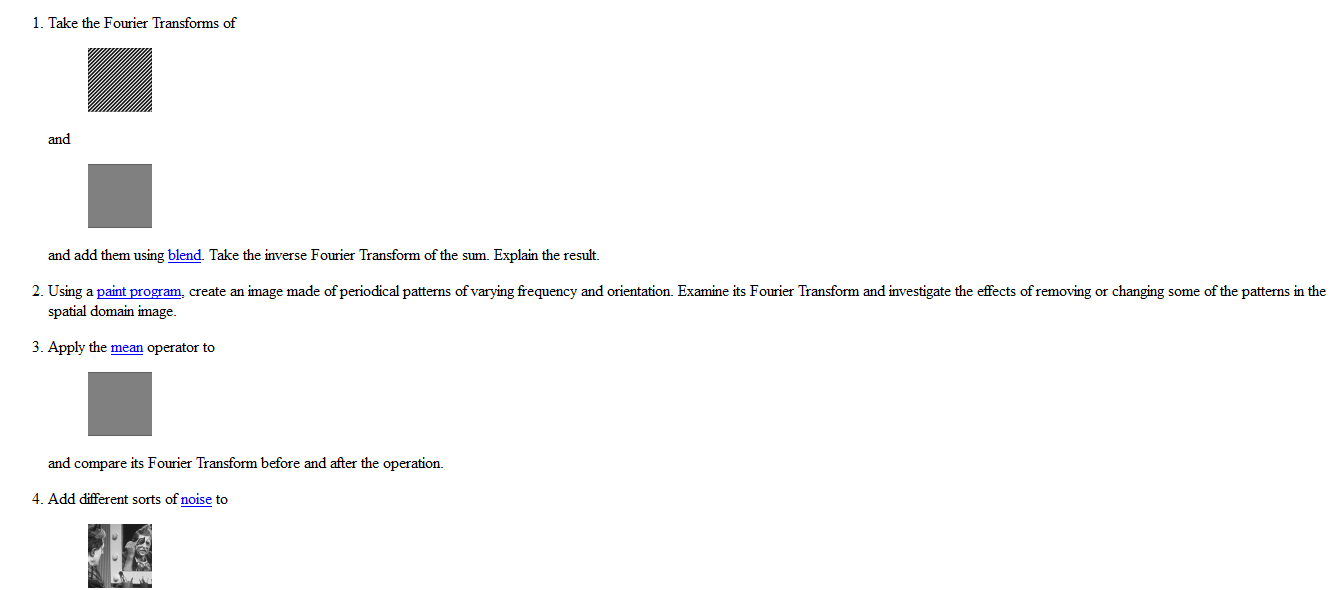
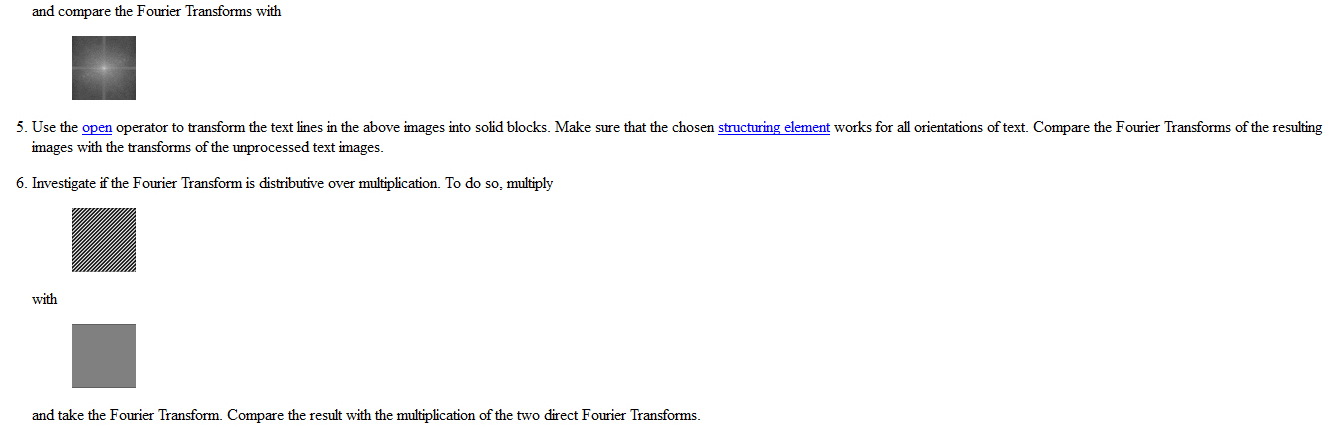